Viewpoint: Balancing the opportunities and risks of generative AI :: Insurance Day
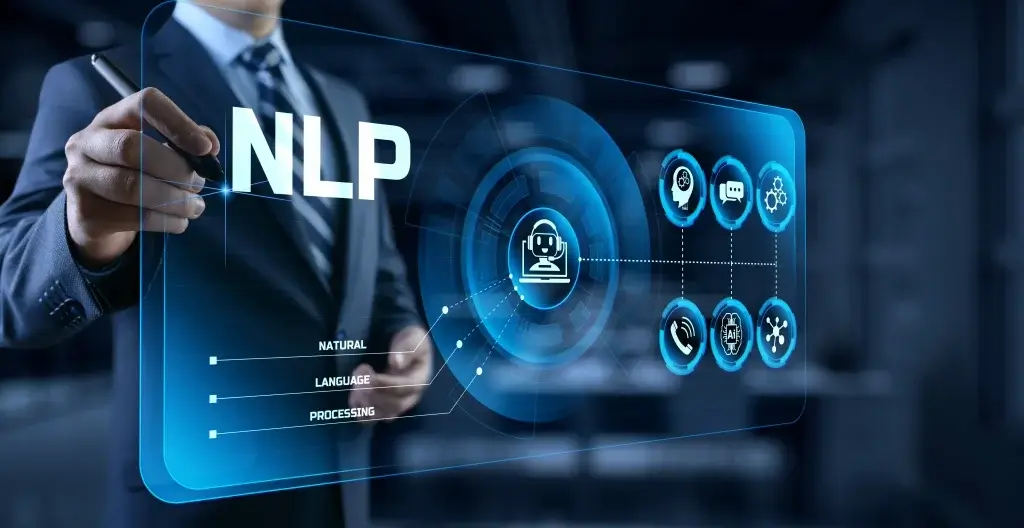
Thanks to these capabilities, AI offers insurers significant benefits, including increased efficiency, cost savings, enhanced productivity, and improved customer satisfaction, engagement, and retention. Recent developments in artificial intelligence (AI), for instance, wouldn’t be possible without the data on which AI is based. In addition, Gradient AI offers automation solutions, such as Gradient Agent Foundry, designed to automate various manual processes within enterprises, allowing users to input task objectives and relevant data. The system then processes this information and integrates into the enterprise’s existing data systems. “We’ve seen it all across the U.S. – climate change is accelerating faster than the insurance industry can adapt, and businesses are paying the price,” said Mike Gulla, CEO and Co-Founder of Adaptive Insurance.
Get additional complimentary resources, including webinars, on the Predictions 2025 hub. Clients can also connect with me through an inquiry or guidance session to discuss topics related to the future of insurance. The total capital raised by COVU now exceeds $20m, including an additional $4m in debt and equity financing to be unlocked once key milestones are achieved. Greg Cole, Head of Claims, AND-E UK explains some of the initiatives that are moving the dial on customer-centric service. Download the report to equip yourself with the knowledge to thrive in this new era of insurance.
Artificial intelligence (AI) promises to supercharge productivity, improve customer experience and drive new business models, but the limitations and risks that come with technology have also come under the spotlight. The generative AI journey holds ChatGPT the promise of unlocking new dimensions in risk insights, operational efficiency, and innovative solutions. However, it is our goal to steer this transformative technology towards a future where AI augments human knowledge for the greater good.
The company will use mea’s GenAI-powered platform to automate and accelerate inbound submission management, significantly reducing the need for manual interventions. Early adopters are already seeing benefits in key customer satisfaction metrics compared to insurers not using GenAI, according to IBM. Per the study, investments in generative AI are expected to surge by more than 300 percent between 2023 and 2025 as organizations move from technology pilots to implementation. IBM’s rivals, including Microsoft, have bet big on such a move, although analysts and traders have hinted at the AI bubble potentially popping in the not-too-distant future. Initially rolled out in Singapore and Malaysia, MedLM analyses medical reports, invoices, and other claim documents, providing summaries and recommendations to claims assessors, reducing manual data entry and minimising errors. Its user-friendly interface ensures that advisors can engage with clients in a seamless manner, offering personalized interaction at each stage of the process.
Despite the use of AI in a handful of areas and pilots taking place elsewhere, insurance organizations are not gaining an advantage on competitors by using this technology more widely. Doing so could enable businesses to work faster, more flexibly and develop more sophisticated models in response to the evolving market. As AI technology advances, insurers have the opportunity to refine their customer interactions, making them more intuitive and value-driven. Customer service is evolving with real-time updates, omnichannel communication, paperless and automated documentation, and virtual assistants. This transformation extends beyond the insurance industry, as companies embrace innovation to enhance customer experiences. As AI systems take over repetitive and analytical tasks, the human workforce can shift towards roles that require empathy, ethical judgment, and complex problem-solving.
People are the heart of innovation
The main idea is to minimize the errors made by the previous model iteratively, thereby improving performance. Agentech, a leading AI-powered workforce solution provider for insurance claims, has successfully raised $3m in seed funding within 30 days. Investment in data analytics within the insurance industry during 2024 to the end of September has grown by 220% compared to the entirety of 2023, a new report has found.

When looking for a financial lines insurance partner, sustainability should be top of mind. Knowing that an insurer can provide solutions in a hard market alongside the soft will not only provide peace of mind but also help build resilience. Leaders are often tasked with making difficult decisions — sometimes with limited data — and communicating these decisions with confidence while acknowledging their inherent uncertainty. “The breakneck speed of Al advancements has the world and our industry running to keep pace. While regulations continue to grow and develop regarding Al usage in insurance, there are several things we already know to be true. Regulators are increasingly advocating for enhanced transparency and accuracy in how insurers assess risk,” the survey noted.
Why is the insurance industry stuck?
This naturally makes gen AI a great fit to augment our work, as the technology can be used to sort through huge quantities of data, extract any relevant information and be even more precise in how we analyze risk. It can also free up much of the time spent on repetitive tasks, making use of people’s expertise more efficiently and further tailoring the support and guidance we provide to our customers. Insurance premium modeling plays a crucial role in setting fair, accurate and competitive premiums in the industry. Actuarial teams, who specialize in risk management, use these models to predict the right premium to charge customers while balancing profitability with market competitiveness. As AI solidifies its position in the auto insurance industry, it is vital to foster a continuous and open dialogue among all stakeholders—insurers, regulators, technologists, and consumers.
- It also brings together insights from KPMG professionals and industry leaders from Generali Italia, PassportCard, Prudential and Zurich Australia who share their perspectives on how to unlock the technology’s full potential.
- Learn how insurance companies create a better employee experience by offering a flexible work environment.
- Using the data, insurers can better assess risks and increase operational efficiencies.
- Gradient boosting machines (GBMs) are a powerful ensemble learning technique that builds a model incrementally by combining weak models (typically decision trees) to form a strong predictive model.
This collaborative approach will be instrumental in achieving balanced AI adoption, ensuring that innovation is pursued thoughtfully with ethical considerations at the forefront. A significant proportion of global consumers would be happy for an artificial intelligence (AI) tool to determine the outcome of their claim, according to a GlobalData survey. As AI adoption continues to gain traction, insurers must make sure their solutions are able to deliver on the customer experience as tolerance for failure will be limited. It is important, however, to ensure transparency in the use of AI for decision-making processes. By clearly communicating how AI is used to make decisions, insurers can build trust and ensure customers understand how their information is handled and how decisions are made.
AI integration is essential not just for current compliance but also for future-proofing the insurance industry. AI systems can quickly adapt to evolving regulations, ensuring ongoing compliance without extensive manual adjustments. Queen pointed out that while automation is beneficial, the most complex and judgement-based tasks in captive insurance will remain out of reach of AI for the foreseeable future. This reality limits the full potential of AI in the industry, although it underscores the importance of human expertise in areas such as claims management and underwriting.
Exclusive: Hacker uses Telegram chatbots to leak data of top Indian insurer Star Health – Reuters
Exclusive: Hacker uses Telegram chatbots to leak data of top Indian insurer Star Health.
Posted: Fri, 20 Sep 2024 07:00:00 GMT [source]
KPMG professionals are working closely with the insurance business to consider how an AI-based solution will enable customers to simply ask a virtual assistant question like “what is my life insurance coverage? This provides a cost-effective way to answer queries the first time, while reducing call volumes and improving customer satisfaction. Waiting times for queries that require human input will likely be reduced and customer service agents can focus on customer queries that require human input. Similar to the ‘happy path’ concept, more routine claims can be partially or even fully automated. This frees handler resource to deal with more complex cases, improving operational efficiency, as well as enhancing the customer experience by reducing waiting times and improving the accuracy of decisions.
As such, insurers must make sure that the rollout of their AI solutions, including AI-powered bots or digital avatars, is optimized to deliver the right experiences at the right time. The optimism held by the majority of insurance insiders reflects the belief that AI’s development is still in the early stages. Many within the industry see the potential for AI to become a driving force in transforming core functions, such as the automation of claims processing and underwriting decisions. The ability to handle large volumes of data in real time and provide insights based on patterns that human analysts might miss is seen as a way to significantly streamline operations and reduce errors.

Companies like Axa, Allianz, Tata, and Audi use Roadzen to provide a better auto insurance experience to every driver on the road. Previously, Mr. Malhotra served as the Chief Executive Officer of Avacara, an enterprise software and data analytics company that provided product development services to Fortune 500 companies. Mr. Malhotra holds a bachelor’s degree in engineering from NSIT, Delhi University, India and a master’s degree in electrical and computer Engineering from Carnegie Mellon University where he studied AI and robotics. By analysing patterns and anomalies in data, AI-driven systems are better equipped to detect fraudulent claims, leading to reduced losses and enhanced claims management processes. This is particularly beneficial for captive insurers, as they can streamline operations, save costs, and focus their resources on more value-added tasks.
Given these caveats, many applications will necessitate an AI-assisted approach to scenario development. This process includes sense-checking and adjusting scenarios for specific business use cases, as well as translating narratives into measurable business impacts. LLMs should therefore be viewed as tools to assist with the heavy lifting of generating scenario narratives, rather than a turnkey solution.
We took several steps to make this happen and we encountered many challenges along the way. Before AI was applied, it took our controllers about six months to complete the assessments and produce a report of our insured assets and values. Get breaking news, exclusive stories, and money- making insights straight into your inbox. Rayne Morgan is a journalist, copywriter, and editor with over 10 years’ combined experience in digital content and print media.
IBM: Insurance industry bosses keen on AI. Customers, not so much
With an impressive 350-year legacy, MSIG USA is doing just that for its clients, utilizing its global presence to further its clients’ goals. Leaders want to know that, at the end of the day, their business is a priority for their partners. At MSIG USA, “we aim to be a stable and sustainable presence for our clients,” Guild said. Two recent One Call hires demonstrate the positive effect external talent and perspectives have on bringing forth successful workers’ compensation solutions.
The Earnix report highlights insurers’ struggle with legacy infrastructure that hinders collaboration and innovation, with 47% of executives citing siloed systems as a significant impediment. Compliance pressures continue to grow, with insurers worldwide prioritising regulatory investments. European and Australian insurers report heightened compliance concerns, driven by strict regulations such as Solvency II.
Pool Re appoints Stark as head of underwriting
Furthermore, many agreed that AI is important for the future of the insurance industry with 80% saying it is enabling new avenues of profitable growth and 73% stating that carriers who adopt AI will outcompete those that do not. In addition, 73% of insurance leaders also believe AI models will help to manage climate-related losses. Only 20% considered AI and machine learning models to be the most accurate, but 27% of respondents believed that a combination of different models offers the best risk prediction. “For us, this is all about improving this integrated experience between health insurance, prevention, and access to medical care,” Alan co-founder and CEO Jean-Charles Samuelian-Werve said. Alan has long offered its customers a chat interface that lets them ask a question to a doctor and get an answer within 15 minutes or so. The next logical step these days would be to leverage artificial intelligence for medical conversations, so Alan is adding a virtual assistant called Mo to the chat feature.
You can foun additiona information about ai customer service and artificial intelligence and NLP. This expanded partnership will enable AXIS to streamline key processes, particularly in submission clearance, and improve customer service delivery across its markets. GlobalData’s 2024 Emerging Trends Insurance Consumer Survey found that 39.2% of consumers around the world would be comfortable or very comfortable with an AI tool to decide the outcome of an insurance claim they have made. Making a claim is often one of the most stressful points at which policyholders interact with insurers, if not the most stressful time.
The industry’s exploration of this technology is marked by a cautious optimism, recognising the need for a balanced approach. Concrete use cases are being tested, aligning with regulatory requirements and internal standards to ensure responsible and ethical deployment. The collaboration aims to leverage Akur8’s Core Platform solution to enhance LWCC’s predictive modeling framework, thus driving high-quality chatbot insurance underwriting insights. This move is designed to bolster the precision and efficiency of LWCC’s pricing processes, aligning with their ongoing growth initiatives and their dedication to innovation. This integration enhances the company’s core insurance offerings by embedding intelligence into their software, allowing insurers to automate tasks, improve decision-making, and deliver data-driven insights.
There is prolonged downtime and data loss for numerous tech firms, with insured losses from business interruption and equipment replacement exceeding US$150 billion. We can foresee a nearfuture of more accurate diagnostics even with complex risks such as climate change and we aim to use the data available to adapt our process. Other than making this a routine part of our property insurance process we will also be applying AI technology to our risk assessment of climate-related exposures. This year represents the first time we’ve applied artificial intelligence to our property insurance process to calculate our insured assets – with great results. The traditional claims process can be notoriously slow, burdening claims adjusters with reams of claims to manually review in a limited amount of time.
Eventually, we might even see AI-generated catastrophe models capable of simulating probabilistic losses. The potential applications are as vast as they are exciting, and our engagement with this technology can unlock the door to new capabilities in catastrophe risk assessment. The data collection and processing required for AI-driven decision-making can lead to potential breaches and misuse of sensitive information. Therefore, insurers must implement comprehensive data security practices to minimise these risks and maintain customer trust. Allianz Trade’s work in TCI involves gathering and analyzing large amounts of financial and extra-financial information in order to assess credit risk and give our customers the appropriate coverage for their transactions. In fact, our database includes credit risk grades on around 83 million companies around the world.

Our team can help you dight and create an advertising campaign, in print and digital, on this website and in print magazine. Many areas of the industry, particularly those requiring human judgement, are simply too complex for AI to take over. Instead, AI can free up professionals’ time by automating mundane tasks, allowing them to focus on higher-value activities. He also acknowledged the significance of the BMO Insurance launch, emphasizing its role in enhancing the insurance experience for advisors and clients. Barry added that Microsoft looks forward to continuing its collaboration with BMO to drive innovation in the industry.

Many insurers have already started introducing machine learning (ML) or other AI technologies to help improve specific business processes, such actuarial models and fraud prevention processes. Cake & Arrow is an experience design and product innovation company that works exclusively with the insurance and financial services industries. Our human-centered design approach helps carriers, distributors, and insurtechs create transformative digital experiences that drive results. Since 2002, Cake & Arrow has partnered with leading insurance and finance companies, including MetLife, Aflac, Citigroup, Travelers, Chubb, Amwins, and The General.
Advances in computer vision and telematics promise improvements in accident prevention and driving habits, resulting in fewer claims and reduced costs. These applications are making the mobility ecosystem smarter, faster, more transparent, and efficient by improving road safety. Through real-time alerts to prevent accidents, which lowers claim frequency and severity, both insurers, customers, and the larger society benefit.

For underwriting, particularly in property and casualty insurance, GenAI can extract critical information from submissions, helping underwriters assess risk more effectively and make faster decisions. The insurance industry thrives on data—much of it unstructured, complex, and dispersed across various platforms. GenAI excels at processing this type of information, making it invaluable for enhancing operational efficiency and ChatGPT App customer engagement. While some companies have begun deploying GenAI for tasks like claims processing and underwriting automation, they’re often missing the bigger picture. To truly harness the transformative power of AI, insurers need a comprehensive strategy, that goes beyond isolated applications. Embracing ecosystems and platforms can help insurers adapt to market changes and even reduce the risk market disruption.
At WTW, we have been refining this practice to aid our insurance clients in developing a broad range of scenarios relevant to their exposures. Furthermore, whilst using LLMs helps to avoid introducing human cognitive biases, scenarios produced by generative AI may inadvertently reflect biases present in their training data or model code. And while LLMs can produce scenario narratives, they cannot currently do the quantitative bits very well, such as estimating losses or evaluating business impacts. However, before turning to your favorite LLM, it’s important to note the difference between AI-generated scenarios and AI-assisted scenario development. Although the earthquake scenario provided above is plausible, this is not always the case.
Sustainability is proven in an insurer’s ability to come through on the promises it makes. For example, MSIG USA’s approach has earned it an A+ rating, proof that its team can back the promises it makes and see them through to completion. “We are confident in our ability to serve your insurance needs, on a global scale,” Guild added. Below are several qualities to look for in a partner that has the experience and insights to help mitigate and navigate their insureds’ unique exposures, giving leaders the space to focus on their core operations. This push for transparency extends beyond internal operations, with 79% of executives advocating for regulatory mandates requiring model transparency. When it comes to the perceived accuracy of these models, there’s a notable lack of agreement on which type of model is most effective for predicting risk, the ZestyAI study found.